Wear particle analysis is one of the main indicators for the detection of wear condition. Traditional computer-aided wear particle analysis employs a unidirectional linear process, i.e. image preprocessing→wear particle segmentation→feature extraction→wear particle recognition→wear condition detection. It has disadvantages such as inaccurate segmentation of wear particles, numerous steps in the “artifcial design feature method”, accumulation and transmission of errors, and difculty in overall optimization of the algorithms. In this study, a framework from overall determination to a detailed recognition is proposed to realize intelligent ferrography analysis. Furthermore, a convolutional neural network (CNN) is developed to classify seven types of ferrograph images, which can be used to directly determine wear conditions including initial wear, normal wear, abnormal wear, and severe wear. Compared with the traditional methods, the proposed CNN can automatically extract features layer by layer, yielding accurate end-to-end classifcation of the ferrograph images. The average accuracy of the CNN model on the test set is 90%. This research provides an efective solution for the automatic identifcation of wear conditions.
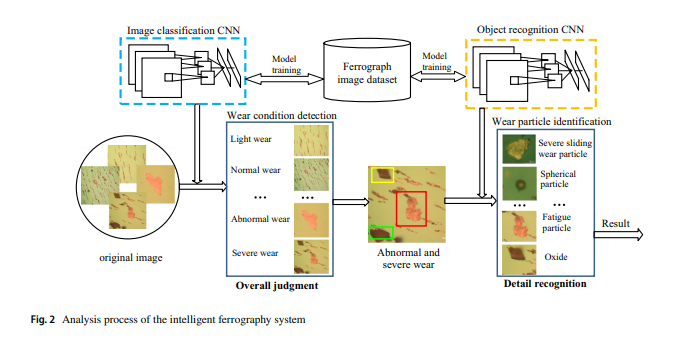
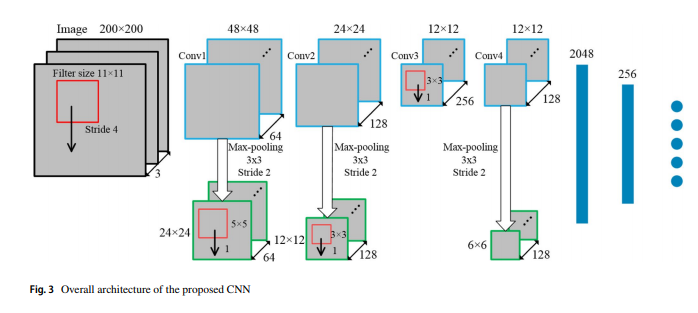
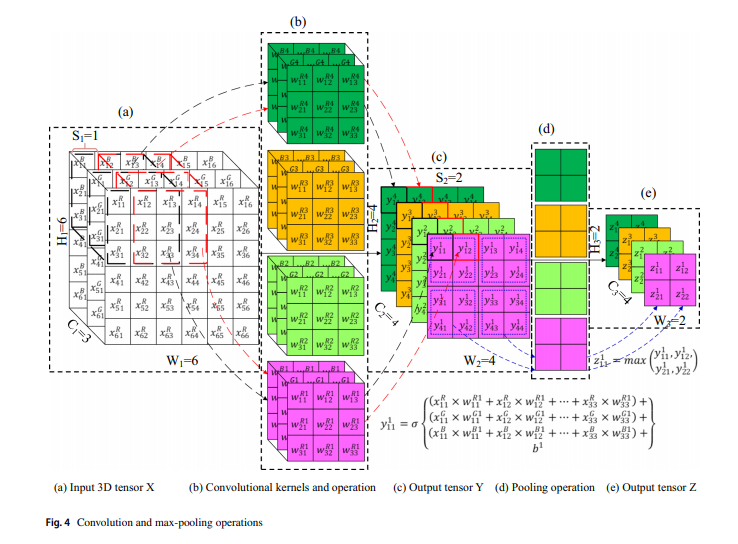
Direct detection of wear conditions by classifcation of ferrograph images.pdf